Communicating Science: The "significance" of Statistics
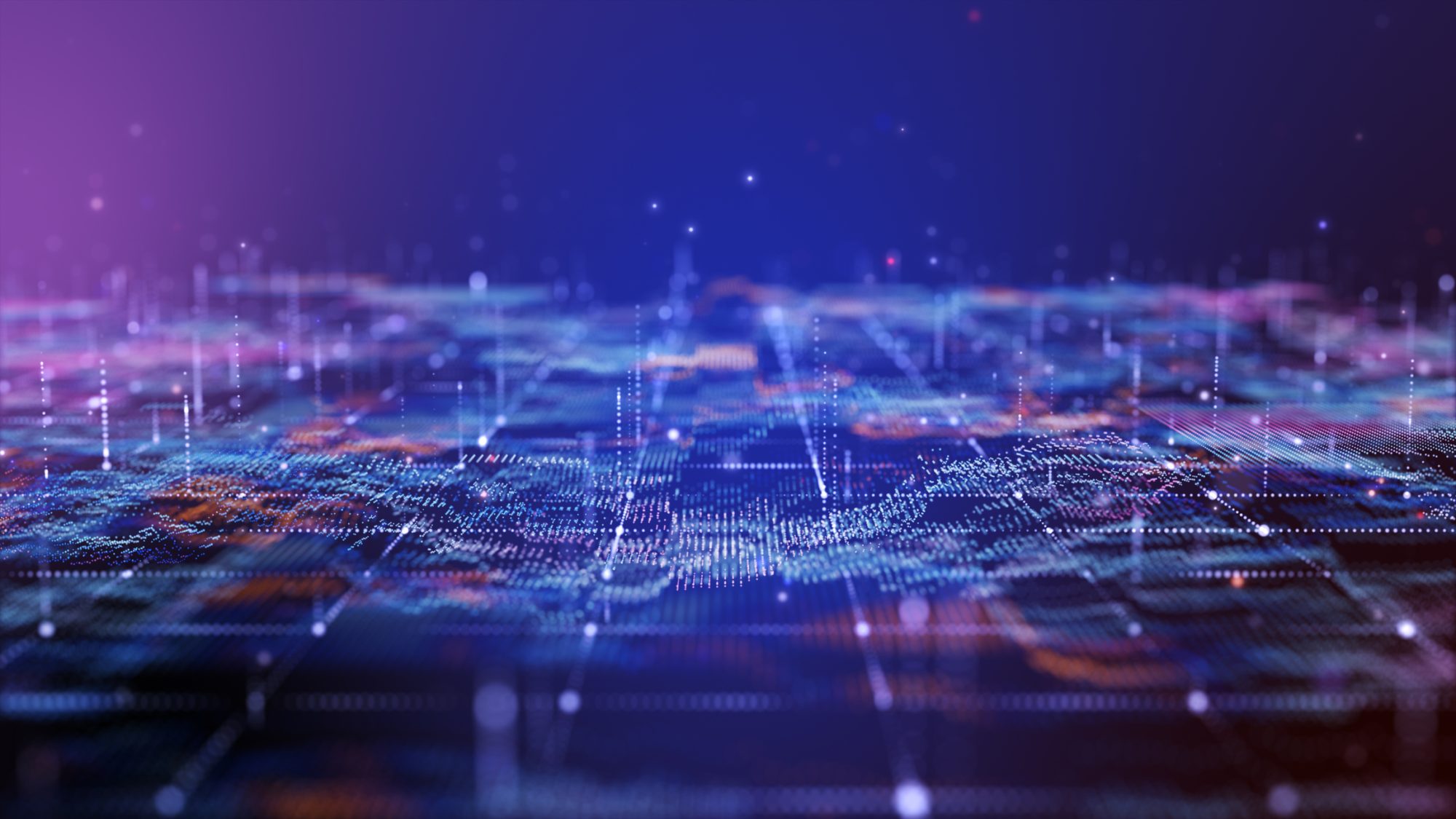
Send us a link
Horizon Europe is heading into its third year, and the latest statistics offer a glimpse of how the EU is spending its €95.5 billion research funding pot.
Any single analysis hides an iceberg of uncertainty. Multi-team analysis can reveal it.
Adopting behaviors of people who buck trends could boost public health and sustainability. In any large dataset involving the choices people make, a handful of people will succeed when most others like them fail. Zooming in on those outliers and mapping out how they made their choices could give those failing in similar circumstances a leg up.
Studies and surveys confirm that during the COVID-19 pandemic, women's workload at home has increased. Does that mean women researchers are also submitting fewer proposals to the SNSF? Analyses show that, with one exception, their share has remained stable.
As the pandemic worsened in the United Kingdom during spring 2020, political disputes turned in a strange direction. The UK government started to claim that the UK’s Covid-19 statistics could not be compared with any other country.
It's human nature to spot patterns in data. But we should be careful about finding causal links where none may exist, says statistician David Spiegelhalter
Sampling simulated data can reveal common ways in which our cognitive biases mislead us.
How a small university team at Johns Hopkins built a COVID-19 data site that draws 1 billion clicks a day.
Up-to-the-minute reports and statistics can unintentionally distort the facts.
Here's what the oft-cited R0 number tells us about the new outbreak-and what it doesn't.
Whether or not "the foundations and the practice of statistics are in turmoil",1 it is wise to question methods whose misuse has been lamented for over a century.
Earlier this fall Dr. Scott Solomon presented the results of a huge heart drug study to an audience of fellow cardiologists in Paris. The presented number 0.059 caused gasps as the audience was looking for something under 0.05.
What can authors and reviewers do to keep common statistical mistakes out of the literature?
An examination of introductory psychology textbooks suggests that prospective psychological researchers may learn to interpret statistical significance incorrectly in their undergraduate classes.
While statistical significance sends the so-called significant results into the literature, the results on the other side of the threshold often disappear into the “famous file drawer”.
"Today I speak to you of war. A war that has pitted statistician against statistician for nearly 100 years. A mathematical conflict that has recently come to the attention of the ‘normal’ people."
For years, scientists have declared P values of less than 0.05 to be "statistically significant." Now statisticians are saying the cutoff needs to go.
Statisticians are calling on their profession to abandon one of its most treasured markers of significance. But what could replace it?
Looking beyond a much used and abused measure would make science harder, but better.
When evaluating the strength of the evidence, we should consider auxiliary assumptions, the strength of the experimental design, and implications for applications. To boil all this down to a binary decision based on a p-value threshold is not acceptable.
P-values and significance testing have come under increasing scrutiny in scientific research. How accurate are these methods for indicating whether a hypothesis is valid?
John Ioannidis discusses the potential effects on clinical research of a 2017 proposal to lower the default P value threshold for statistical significance from .05 to .005 as a means to reduce false-positive findings.
A study has revealed a high prevalence of inconsistencies in reported statistical test results. Such inconsistencies make results unreliable, as they become “irreproducible”, and ultimately affect the level of trust in scientific reporting.
Statistical significance and hypothesis testing are not really helpful when it comes to testing our hypotheses.
Reproducible research includes sharing data and code. The reproducibility policy at the journal Biostatistics rewards articles with badges for data and code sharing. This study investigates the effect of badges at increasing reproducible research, specifically, data and code sharing, at Biostatistics.
A new paper recommends that the label “statistically significant” be dropped altogether; instead, researchers should describe and justify their decisions about study design and interpretation of the data, including the statistical threshold.